With the explosion of terms like Artificial Intelligence (AI) and Machine Learning (ML) in construction, AEC Magazine caught up with Senthil Kumar, CTO and head of AI at start-up Slate.ai to discuss how the technology can assist the complexity in the building industry
Q: Senthil, it’s always great to meet a new face in AEC software. Can you tell us about your previous work and what you do at Slate?
A: I’ve been working on advanced data technology in global software companies for over two decades now. I’ve helped pioneer multiple technological endeavours during that time, including AI, Blockchain, dge, cloud computing, metaverse, IoT, swarm robotics, system autonomy, and Big Data computing in industries spanning autonomous vehicles, fintech, smart buildings and cities, geospatial engineering, insurance, health care, and medicine.
I’ve also helped develop the emerging AI Industry standards, such as the EU Artificial Intelligence Act, and also work in numerous industry think tanks and advisory boards of well-known academia and startups. Recently, my pioneering AI work was cited in the wall street journal and was recognised by the World Economic Forum.
I joined Slate 12 months ago as CTO, to build a new team of engineers and data scientists focused on delivering the most modern software and technology approaches to leverage data across building production.
Q: AI and Machine Learning are often greatly misunderstood. From your point of view, explain what they are?
A: I agree these terms have been misused interchangeably. Artificial Intelligence (AI) is a branch of computational science that enables the creation of smart intelligent machines and systems. AI offers the promise of a bridge between humans and machines in smarter ways, mimicking human cognition and enabling the creation of a smarter harmonious ecosystem interplay by understanding a human’s needs and intent.
Now, while AI is a broad science, machine learning is a specific subset of AI that trains a machine on how to learn and helps one build AI-driven applications. In a sense, ML is how computer systems learn and develop their intelligence.
Q: Does that mean you can have AI without Machine Learning?
A: Yes, as much as it may surprise us, we can have AI without ML. While model training, ML methodologies, and selflearning techniques have grown exponentially in recent times, there are aspects of AI that can be on their own without the need for ML to have taken place. An early oft-used method of AI implementation was the use of Decision Trees that break down complex problems into binary evaluations, then traverse those trees to find answers. This is not ML dependent and is widely used to this day.
Other examples of AI that are not ML-dependent include, using machines to solve shortest path problems, constraint solving, chatbots, and aspects of NLP [Natural Language Processing] using stochastic grammar to parse sentence trees. We have to remember that AI is vast in scope and ML is just one of the many techniques that AI uses.
Q: So, from your experience, what are some other common misconceptions about AI?
A: Don’t get me started! I think we’ve all heard that AI systems will probably replace our jobs, making most people redundant. This is a myth that’s heard to this day. Similar arguments have been made during the agrarian and industrial revolutions. The reality is that AI will mostly change existing jobs and create new ones. We have to remember, while on the one hand, a number of jobs might be automated by AI, the main reason that will occur is because those jobs are generally well suited to the way a computational machine works.
Roles that are best suited to human intellect and the supervision systems will continue to thrive and grow. The migration of work from repetitive physical roles towards cleaner, more rewarding careers, that humans are brilliantly well-suited to, started a long time ago, way before we were talking about AI. The overall quality of life and our productivity will only become better with a harmonious Human-Computer interplay, leveraging what machines do well in partnership with what humans do well.
Another misconception is that AI systems can be more impartial than human beings and be objective.
For some time now, creating unbiased and accurate AI has been the aspirational Holy Grail of AI architects. Even though AI systems do not yet have emotional intelligence like humans, they are susceptible to influences. It is imperative to note that AI is based on inputs and inferences from human experts and sometimes fallible data.
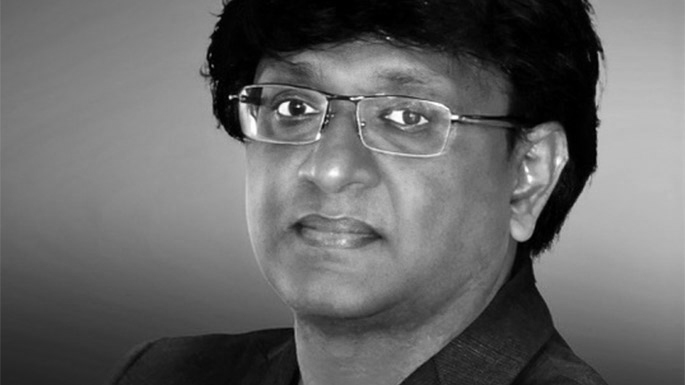
Since humans are intrinsically biased in one way or another, so is the AI that has been co-created by humans. These biases can and do get carried over to the systems. A particular issue in the news is the systems that self-train using data from social inputs. They can be extremely susceptible to unintentional or intentional malevolent influences and biases. For example, the early facial recognition systems had biases in identifying ethnicities, and other systems that used social feeds such as twitter were unduly influenced by false propaganda. While technology implementations for AI are advancing swiftly and ethics, particularly for autonomous AI, is improving, we still have a long way to go in creating truly impartial and objective AI systems.
Q: Why did you see building production as the next best place to implement what you’ve learned?
A: While technology has transformed the landscape in several industries, there are aspects of building production that are yet to be touched by technological advancements that have already proved valuable elsewhere. Years ago, in industries such as retail, healthcare, insurance and fintech, we’ve been able to bring about significant changes to improve overall efficiencies, implementing both simple and complex AI implementations.
I joined Slate when I saw that building production is a multi-trillion-dollar industry that impacts the economy in a multitude of ways. Here I can leverage my knowledge of these technologies to create a meaningful and significant altering of the value-map for building.
If we think about this, improvements in construction can have a direct impact on the global economy. The interdependencies in building production are inherently global in scope, involving supplies, technologies, and participants across different countries, making this a truly global phenomenon. No other industry of today presents such a diverse array of unsolved challenges, and I believe many of those challenges can be solved more easily than imagined.
Complexity and unforeseen challenges are the norms in building production. Every aspect of this environment that flexes human and machine intellect is exciting to me, allowing us to employ some of the latest technologies and ideas in a meaningful manner. Just a few examples include: supply chain, and schedule optimisation utilising some of the major advancements in AI, computer vision, and cognitive sciences; Metaverse Augmented Reality applications for digital simulation and digital rehearsals; the ability to predict cost overruns and anticipatory analytics to illustrate when stages of building can be completed.
The interdependencies of construction’s inherently multidisciplinary, multi-dimensional and multi-player ecosystem makes it incredibly interesting [for AI], but also well suited to modern computational solutions
I’m also excited see new ‘smarter’ materials which complement building performance, utilising advancements in polymer science and computational nanotechnology, with multi-dimensional analytical tools that can leverage their potential to reduce carbon in operational performance. What other industry would offer the multitude of challenges this industry poses? All of these are exciting to me as they relate to building production.
Q: AI and chess have become synonymous with explaining what AI can achieve. So, is construction more complicated than, for example, chess?
A: Ah, yes… I might argue that elements of construction are a more complex challenge than a chess game. In a chess game, the player anticipates the moves of the opponent. Not just the next move but several moves ahead of what the opponent may do. This was generally viewed as the peak of challenges to solve with AI. That’s why when [chess computer] Deep Blue beat a human in a chess game there was elation all around.
But, if we think about this, chess has a singular opponent, the game board is defined, the rules are defined. Even though the number of moves can be huge, there is still a finite number of moves an opponent can make with the game pieces. Construction, on the other hand, has inherent complexities. Think of it as a game with an infinite number of opposing moves, multiple opponent players teaming up in unison against the player.
Some of the moves may not even be fully well defined, such as unforeseen political events, supply constraints, surprise government regulations, workmanship constraints. All of these can throw a wrench into the well-designed construction schedule. This can make it significantly more complex than a game of chess.
Q: So, how complicated are the problems in building production? How does the complexity compare with other industries, such as health, finance, and biotech?
A: Well, building production is a daunting challenge. From conception to completion for the comfort and safety of human occupants and operational efficiencies, improving building production is among the most sophisticated and complex legacy industries to improve with technology.
While tremendous advancements have been made in healthcare, manufacturing and finance, the difference between those industries and construction has been the pace of innovation and adoption. The interdependencies of construction’s inherently multi-disciplinary, multi-dimensional and multi-player ecosystem make it incredibly interesting, but also well suited to modern computational solutions. It’s incredible for me to see how many construction decisions are made without the situational context commonly available in other industries from well understood dimensional analysis techniques.
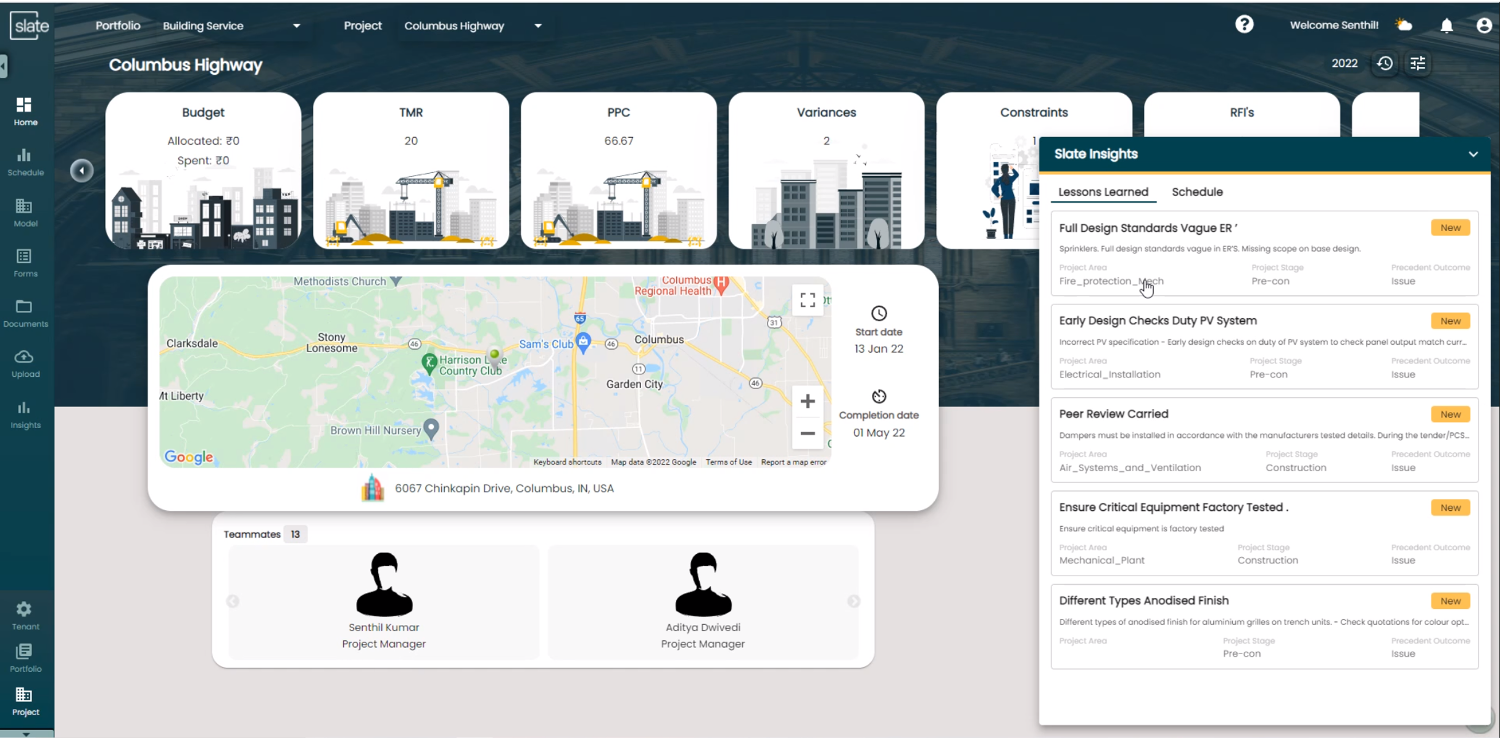
To improve the Decision Value Chain in building production, multi-dimensional analysis can be a very effective strategy. This type of Analytical Reasoning involves data segmentation and contextualisation in varying dimensions.
In the case of building production, a number of challenges can be analysed in cross-sectional contextual patterns of varying dimensions. Real world events across space and time can be correlated into contextual dimensions – causation analysis, co-occurrence patterns of features with events and values of importance.
It could be as simple as computing the optimal time for performing certain construction tasks, or how should a specific work package be formulated and in what sequence should certain events be orchestrated? The ability to foresee and foretell events before they happen as it relates to construction can all benefit from multi-dimensional analysis to improve the decision value chain as it relates to building production.
I was recently asked whether going to Mars is more challenging than construction. For me, I might contest that building production and sustainability on this planet and on Mars when we get there are the more interesting goals.
Q: Where are we in the evolution of AI in construction?
A: We are witnessing the creation of history in our times. Most leaders in construction have recognised that what the 4th industrial revolution did to the invention and patronisation of advanced technologies in traditional industries, the construction spectrum might finally be ready to adopt.
Advancements are being made in a number of AI disciplines, including Convolutional Neuroscience, Realtime Machine Vision, Human-Level Cognition with NLP, intent-based computations, and a favourite focus area of mine, “Speed of Thought” analytics, where, as you think, so shall the machine cater to your intent.
While AI is going mainstream in other markets, adoption of it in the construction space has been lagging. This was not for want of technology or digital data but scepticism around AI, with a bias towards human experience being used as context for most decisions.
Here at Slate, the biggest attitude change we are seeing in construction is happening when organisations are feeling completely overwhelmed with software and data, while not benefiting from the intelligence that the data can provide. Existing software has taken the automation of construction’s legacy workflows as far as they can go. Just knowing how many RFIs you have unprocessed, or how many change orders are stuck is not improving the bottom line.
The current best-in-class software solutions are helping companies try and keep to the schedule and budget frameworks that have not changed significantly for decades. To gain from the digital motherlode of knowledge we are amassing in building production, we need new AI solutions that work with humans before, during and after the critical decisions that can and will improve outcomes, not just maintain current industry performance. We believe our work at Slate will be significant in bringing that value to the industry.
Slate.ai will be attending AEC Magazine’s NXT BLD event in London on 21 June 2022
Source: AEC Magazine