AI Bias: Understanding Its Causes, Impact, and Prevention
AI bias occurs when artificial intelligence systems produce unfair or discriminatory outcomes due to flawed data, design, or implementation. This issue can undermine trust in AI, exacerbate existing inequalities, and result in societal and financial consequences.
AI bias is a mirror for human bias, amplified by the rapid scale at which artificial intelligence operates. Tackling it requires a comprehensive approach, where developers actively work to build systems that minimize discrimination and inequality.
What is AI Bias?
AI bias refers to systematic favoritism or discrimination in algorithmic decisions, often stemming from imbalanced datasets or unintentional developer assumptions. For example, an AI hiring tool trained on biased historical data may prioritize candidates from certain demographics over others.
Senthil M. Kumar says, “Whether we like it or not, all of our lives are being impacted by AI today, and there’s going to be more of it tomorrow.” Slate’s CTO continues to comment how “Decision systems are being handed off to machines — and those machines are biased inherently, which impacts all our lives.”
Causes:
- Biased Training Data: If AI models learn from datasets lacking diversity or reflecting historical prejudices, they replicate those biases.
- Human Influence: Developers’ assumptions can unintentionally embed biases during model design or feature selection.
- Algorithmic Prioritization: Systems optimized for speed or efficiency may unintentionally trade off fairness.
A major contributor to bias is the lack of representational diversity in training data, advocating for proactive fairness measures throughout development.
Consequences:
AI bias can perpetuate discrimination, such as facial recognition systems misidentifying certain ethnic groups or credit scoring algorithms denying loans to specific demographics. “Bias harms individuals and undermines trust in AI solutions, slowing adoption and innovation.”
How to Address AI Bias
- Use Diverse Data: Building datasets that represent diverse populations helps mitigate bias.
- Conduct Bias Audits: Regular fairness checks throughout the AI lifecycle can identify and address potential issues.
- Collaborate Across Disciplines: Kumar stresses that AI ethics is a societal responsibility requiring diverse perspectives, not just technical fixes.
Sources
This article is based on original reporting by Ellen Glover. Read the full article here. Curious about Slate Technologies? Check out how are tools work here!
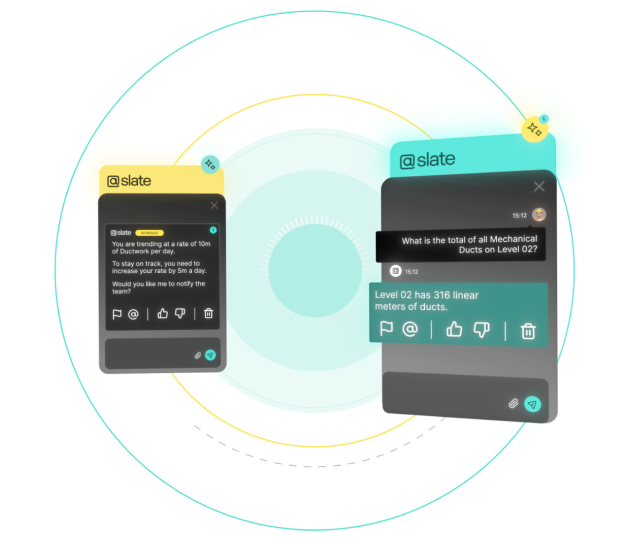